SciWalker
Data intelligence to power discovery
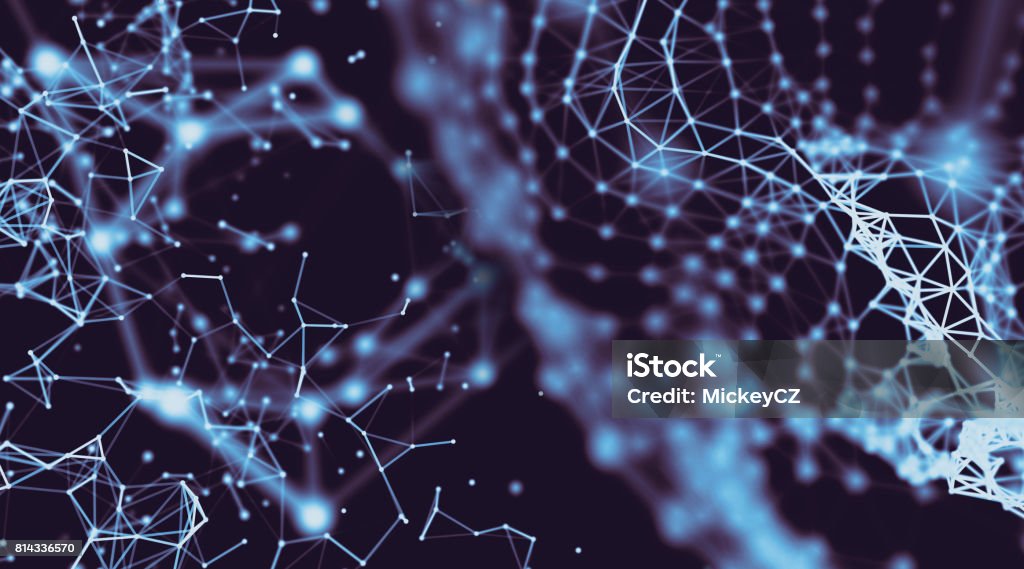
Make data your most vital strategic asset
Unify your research insights and accelerate time to innovation with our semantic metasearch engine, SciWalker.
This customizable end-to-end solution consolidates knowledge fragments scattered across internal and external documents, helping R&D teams uncover hidden connections, find new insights, and drive discovery.
- Search by chemical compounds for associated documents, synonyms, identifiers, properties and parent classes
- Plug in any combination of our ontologies, including one of the largest chemical ontologies
- Merge internal data from multiple formats with fragmented scientific and industrial literature across the life science industry
- Gain immediate analytical insights with built-in tools for relationship extraction and co-occurrence analysis
Choose your relevant data sources, including plugging in Dimensions data that contains
A holistic solution tailored for life sciences
With strong capabilities in the chemistry field, such as chem search
With a variety of available ontologies, including one of the largest chemical ontologies on the market, SciWalker enables complete document mapping, scanning documents, text, images, chemical compounds and structures, applying ontology-driven annotation for easier analysis.
With one of the largest chemical ontologies, including chemical structure, users can search using text or draw a structure to discover all relevant information and similar compounds across documents and extracted reactions - all in a single platform.
Co-occurrence mapping also helps to expose relationships with related concepts like applications or methods.
Normalization
The annotation pipeline starts with a normalization process that homogenizes all kinds of documents from various sources into one format that can then be annotated.
Annotation
All cartridges you selected will be used for annotation. That means the annotator detects domain-specific terms (e.g. chemical terms, diseases or proteins) within the text and marks them for later use.
Extract
Knowledge extraction works by assigning roles to certain concepts that have been marked during annotation and by finding instances in the text that match pre-defined patterns.
Summarize
Summarizing is a process of collecting a wide array of relevant information about a certain topic. This is made easy by using annotated documents.
Search
Ontological search is vastly superior to normal text search. Not only does it recognize the synonyms for the concepts you are interested in, but it also relies on contextual information for deciding more precisely if a term is a protein or just a random abbreviation.
Use SciWalker to expand and refine your search
Ontology-based text search
For example, when searching for 'steroids', automatically find all compounds belonging to this class.
Compound search
Exact, full-match similarity and compound substructures.
Reaction search
Exact, substructure, and subreactions.
Accelerate innovation and discovery
By applying semantic normalization and annotation to you internal and external data, SciWalker makes vital information accessible to teams across your business, saving thousands of hours that would be otherwise spent searching and interpreting results.
Combining some of the industry's most extensive ontologies with semantic algorithms and machine learning enables your teams to advance analysis and discovery.
One multinational pharmaceutical company saved over 800,000 working hours in 12 months.